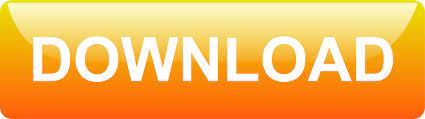
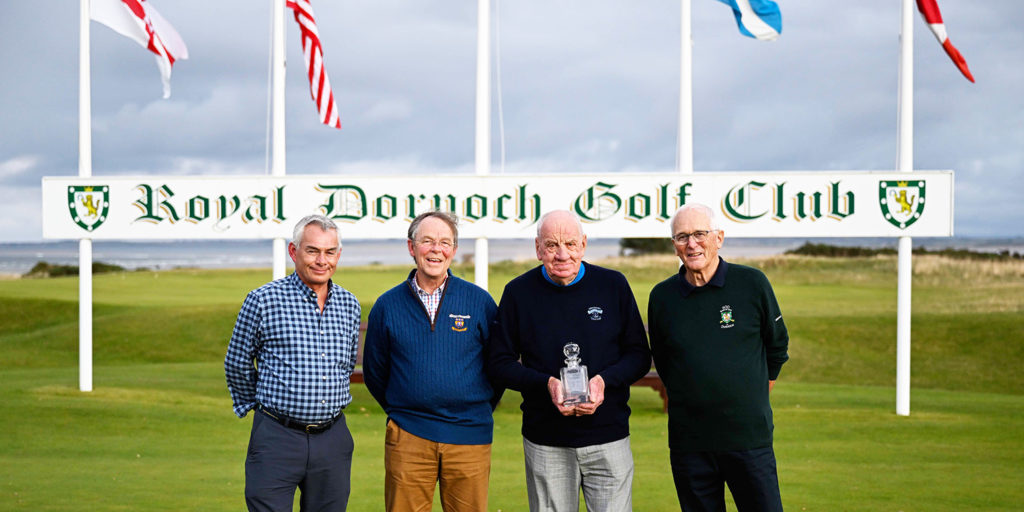
Our current research is focused on several aspects: designing improved machine learning techniques to support automatic labeling of events identifying machine learning techniques in intelligent user interfaces that balance automatic assistance and usability, building innovative interfaces to leverage our activity data, and measuring the impact of such innovative interfaces on reducing costs, errors, and frustration. We have taken a very practical approach, building fully-functional prototypes and deploying them in the wild.
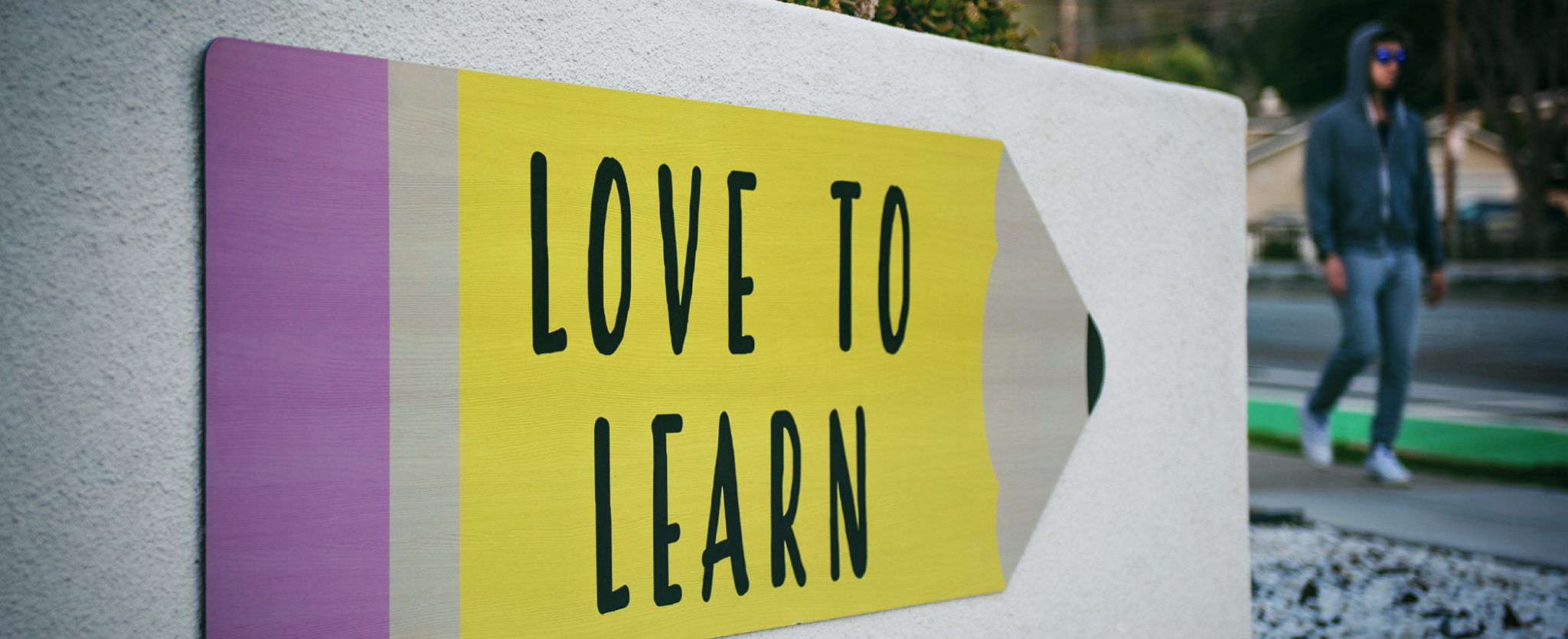
In our research, we are working to demonstrate that our system can help people find their task-related information faster, recover faster and better from interruptions, and maintain better awareness of personal and workgroup tasks. We believe that a database of past user actions on the desktop, segmented by user task, can enable substantial productivity- enhancing features when combined with machine learning. By building software that is aware of those tasks, we can greatly decrease costs, errors, and frustration. The core TaskTracer hypothesis is that people naturally want to organize their information, regardless of data type or storage location, into units that they think of as projects or activities or tasks.

TaskTracer is a research project at Oregon State University to identify innovative technological solutions to information overload and task management at the personal and workgroup levels.
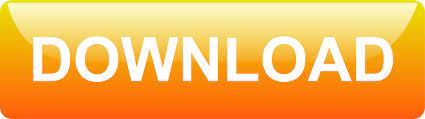